What we are interested in
Machine learning has revolutionised the process of analyzing data and has led to new insights and applications. However, one of the key short comings of this field is its use of black box models which may lack explainability. A key aspect of our research is to develop interpretable machine learning algorithms. We do so by using techniques such as adversarial optimization, sparsity, etc. We also focus on the interplay between learning and optimization by developing new algorithms to train machine learning models and exploting machine learning models to improve the process of optimization. Finally, we also aim to identify the theoretical reasons behind the success of such models.
Members
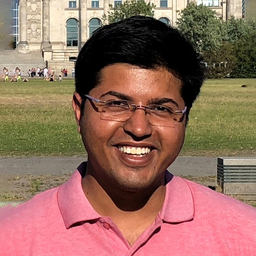
Kartikey Sharma
kartikey.sharma (at) zib.de
kartikey.sharma (at) zib.de
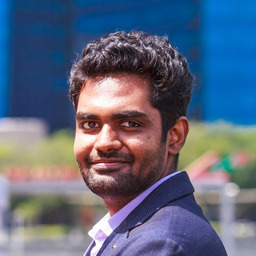
Sai Ganesh Nagarajan
nagarajan (at) zib.de
nagarajan (at) zib.de
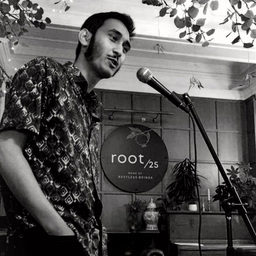
Adnan Mahmud
mahmud (at) zib.de
mahmud (at) zib.de
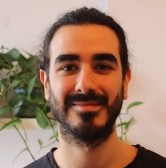
Berkant Turan
turan (at) zib.de
turan (at) zib.de
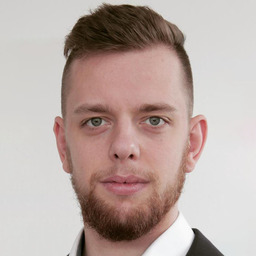
Christoph Graczyk
graczyk (at) zib.de
graczyk (at) zib.de
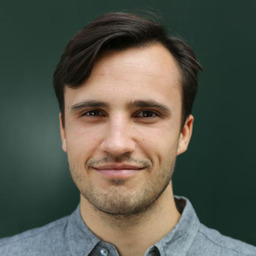
Christophe Roux
roux (at) zib.de
roux (at) zib.de
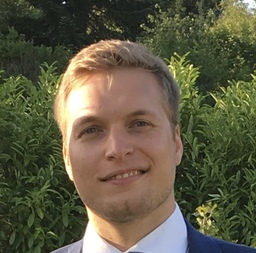
Felix Prause
prause (at) zib.de
prause (at) zib.de
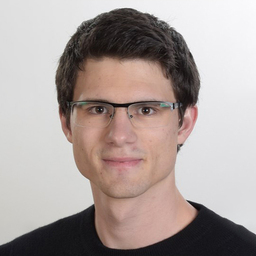
Ingo Meise
meise (at) zib.de
meise (at) zib.de
Kartikeya Chitranshi
chitranshi (at) zib.de
chitranshi (at) zib.de
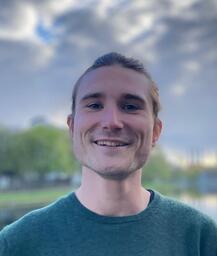
Konrad Mundinger
mundinger (at) zib.de
mundinger (at) zib.de
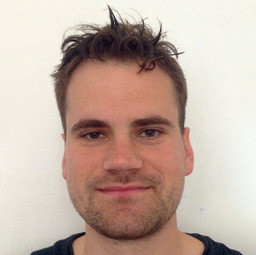
Max Zimmer
zimmer (at) zib.de
zimmer (at) zib.de
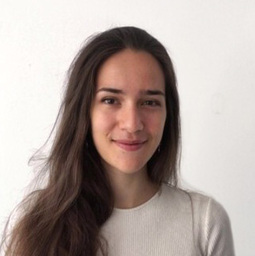
Megi Andoni
andoni (at) zib.de
andoni (at) zib.de
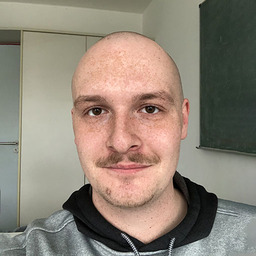
Moritz Wagner
wagner (at) zib.de
wagner (at) zib.de
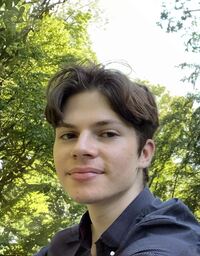
Nico Pelleriti
pelleriti (at) zib.de
pelleriti (at) zib.de
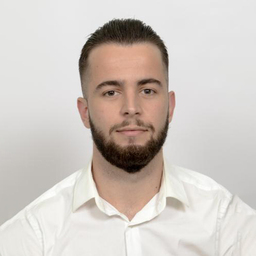
Shpresim Sadiku
sadiku (at) zib.de
sadiku (at) zib.de
Projects
- Adaptive Algorithms Through Machine Learning: Exploiting Interactions in Integer Programming (MATH+ EF1-9)
- Expanding Merlin-Arthur Classifiers: Interpretable Neural Networks Through Interactive Proof Systems (MATH+ EF1-67)
- Learning to Schedule Heuristics in IP
- Globally Optimal Neural Network Training (SPP 2298, project number 463910157)
- AI-Based High-Resolution Forest Monitoring
Publications
- Deza, A., Pokutta, S., and Pournin, L. (2024). The Complexity of Geometric Scaling. Operations Research Letters, 52.
DOI: 10.1016/j.orl.2023.11.010
[arXiv]
[BibTeX]
@article{dpp_geometric_scaling_22, year = {2024}, journal = {Operations Research Letters}, volume = {52}, doi = {10.1016/j.orl.2023.11.010}, archiveprefix = {arXiv}, eprint = {2205.04063}, primaryclass = {math.OC}, author = {Deza, Antoine and Pokutta, Sebastian and Pournin, Lionel}, title = {The Complexity of Geometric Scaling} }
- Göß, A., Martin, A., Pokutta, S., and Sharma, K. (2024). Norm-induced Cuts: Optimization with Lipschitzian Black-box Functions.
[URL]
[arXiv]
[BibTeX]
@misc{gmps_nic_23, url = {https://opus4.kobv.de/opus4-trr154/files/518/nic_preprint.pdf}, archiveprefix = {arXiv}, eprint = {2403.11546}, primaryclass = {math.OC}, year = {2024}, author = {Göß, Adrian and Martin, Alexander and Pokutta, Sebastian and Sharma, Kartikey}, title = {Norm-induced Cuts: Optimization with Lipschitzian Black-box Functions} }
- Wäldchen, S., Sharma, K., Zimmer, M., Turan, B., and Pokutta, S. (2024). Merlin-Arthur Classifiers: Formal Interpretability with Interactive Black Boxes. Proceedings of International Conference on Artificial Intelligence and Statistics.
[arXiv]
[BibTeX]
@inproceedings{wszp_merlinarthur_22, year = {2024}, booktitle = {Proceedings of International Conference on Artificial Intelligence and Statistics}, archiveprefix = {arXiv}, eprint = {2206.00759}, primaryclass = {cs.LG}, author = {Wäldchen, Stephan and Sharma, Kartikey and Zimmer, Max and Turan, Berkant and Pokutta, Sebastian}, title = {Merlin-Arthur Classifiers: Formal Interpretability with Interactive Black Boxes} }
- Zimmer, M., Spiegel, C., and Pokutta, S. (2024). Sparse Model Soups: A Recipe for Improved Pruning Via Model Averaging. Proceedings of International Conference on Learning Representations.
[arXiv]
[BibTeX]
@inproceedings{zsp_modelsoup_23, year = {2024}, booktitle = {Proceedings of International Conference on Learning Representations}, archiveprefix = {arXiv}, eprint = {2306.16788}, primaryclass = {cs.LG}, author = {Zimmer, Max and Spiegel, Christoph and Pokutta, Sebastian}, title = {Sparse Model Soups: A Recipe for Improved Pruning Via Model Averaging} }
- Kevin-Martin, A., Bärmann, A., Braun, K., Liers, F., Pokutta, S., Schneider, O., Sharma, K., and Tschuppik, S. (2023). Data-driven Distributionally Robust Optimization Over Time. INFORMS Journal on Optimization.
[arXiv]
[BibTeX]
@article{abblpsst_distributionally_ro_23, year = {2023}, journal = {INFORMS Journal on Optimization}, archiveprefix = {arXiv}, eprint = {2304.05377}, primaryclass = {math.OC}, author = {Kevin-Martin, Aigner and Bärmann, Andreas and Braun, Kristin and Liers, Frauke and Pokutta, Sebastian and Schneider, Oskar and Sharma, Kartikey and Tschuppik, Sebastian}, title = {Data-driven Distributionally Robust Optimization Over Time} }
- Kruser, J., Sharma, K., Holl, J., and Nohadani, O. (2023). Identifying Patterns of Medical Intervention in Acute Respiratory Failure: A Retrospective Observational Study. Critical Care Explorations.
[BibTeX]
@article{kshn_identifying_23, year = {2023}, journal = {Critical Care Explorations}, author = {Kruser, Jacqueline and Sharma, Kartikey and Holl, Jane and Nohadani, Omid}, title = {Identifying Patterns of Medical Intervention in Acute Respiratory Failure: A Retrospective Observational Study} }
- Thuerck, D., Sofranac, B., Pfetsch, M., and Pokutta, S. (2023). Learning Cuts Via Enumeration Oracles. Proceedings of Conference on Neural Information Processing Systems.
[arXiv]
[BibTeX]
@inproceedings{learn_cut_oracle_2023, year = {2023}, booktitle = {Proceedings of Conference on Neural Information Processing Systems}, archiveprefix = {arXiv}, eprint = {2305.12197}, primaryclass = {math.OC}, author = {Thuerck, Daniel and Sofranac, Boro and Pfetsch, Marc and Pokutta, Sebastian}, title = {Learning Cuts Via Enumeration Oracles} }
- Bienstock, D., Muñoz, G., and Pokutta, S. (2023). Principled Deep Neural Network Training Through Linear Programming. Discrete Optimization.
[URL]
[arXiv]
[summary]
[BibTeX]
@article{principledDNN_LP_2018, year = {2023}, journal = {Discrete Optimization}, url = {https://www.sciencedirect.com/science/article/abs/pii/S1572528623000373}, archiveprefix = {arXiv}, eprint = {1810.03218}, primaryclass = {cs.LG}, author = {Bienstock, Daniel and Muñoz, Gonzalo and Pokutta, Sebastian}, title = {Principled Deep Neural Network Training Through Linear Programming}, summary = {https://www.pokutta.com/blog/research/2018/10/12/DNN-learning-lp-abstract.html} }
- Zimmer, M., Andoni, M., Spiegel, C., and Pokutta, S. (2023). PERP: Rethinking the Prune-Retrain Paradigm in the Era of LLMs.
[arXiv]
[code]
[BibTeX]
@misc{zasp_perp_23, archiveprefix = {arXiv}, eprint = {2312.15230}, primaryclass = {cs.LG}, year = {2023}, author = {Zimmer, Max and Andoni, Megi and Spiegel, Christoph and Pokutta, Sebastian}, title = {PERP: Rethinking the Prune-Retrain Paradigm in the Era of LLMs}, code = {https://github.com/ZIB-IOL/PERP} }
- Zimmer, M., Spiegel, C., and Pokutta, S. (2023). How I Learned to Stop Worrying and Love Retraining. Proceedings of International Conference on Learning Representations.
[arXiv]
[code]
[BibTeX]
@inproceedings{zsp_retrain_21, year = {2023}, booktitle = {Proceedings of International Conference on Learning Representations}, archiveprefix = {arXiv}, eprint = {2111.00843}, primaryclass = {cs.LG}, author = {Zimmer, Max and Spiegel, Christoph and Pokutta, Sebastian}, title = {How I Learned to Stop Worrying and Love Retraining}, code = {https://github.com/ZIB-IOL/BIMP} }
- Kossen, T., Hirzel, M. A., Madai, V. I., Boenisch, F., Hennemuth, A., Hildebrand, K., Pokutta, S., Sharma, K., Hilbert, A., Sobesky, J., Galinovic, I., Khalil, A. A., Fiebach, J. B., and Frey, D. (2022). Towards Sharing Brain Images: Differentially Private TOF-MRA Images with Segmentation Labels Using Generative Adversarial Networks. Frontiers in Artificial Intelligence.
DOI: 10.3389/frai.2022.813842
[BibTeX]
@article{khmbhhpshsgkff_22, year = {2022}, journal = {Frontiers in Artificial Intelligence}, doi = {10.3389/frai.2022.813842}, author = {Kossen, Tabea and Hirzel, Manuel A. and Madai, Vince I. and Boenisch, Franziska and Hennemuth, Anja and Hildebrand, Kristian and Pokutta, Sebastian and Sharma, Kartikey and Hilbert, Adam and Sobesky, Jan and Galinovic, Ivana and Khalil, Ahmed A. and Fiebach, Jochen B. and Frey, Dietmar}, title = {Towards Sharing Brain Images: Differentially Private TOF-MRA Images with Segmentation Labels Using Generative Adversarial Networks} }
- Macdonald, J., Besançon, M., and Pokutta, S. (2022). Interpretable Neural Networks with Frank-Wolfe: Sparse Relevance Maps and Relevance Orderings. Proceedings of International Conference on Machine Learning.
[arXiv]
[poster]
[video]
[BibTeX]
@inproceedings{mbp_interpretfw_22, year = {2022}, booktitle = {Proceedings of International Conference on Machine Learning}, archiveprefix = {arXiv}, eprint = {2110.08105}, primaryclass = {cs.lG}, author = {Macdonald, Jan and Besançon, Mathieu and Pokutta, Sebastian}, title = {Interpretable Neural Networks with Frank-Wolfe: Sparse Relevance Maps and Relevance Orderings}, poster = {https://pokutta.com/slides/20220712_icml_poster_interpretable_rde.pdf}, video = {https://slideslive.com/38983588} }
- Nohadani, O., and Sharma, K. (2022). Optimization Under Connected Uncertainty. INFORMS Journal on Optimization.
DOI: 10.1287/ijoo.2021.0067
[BibTeX]
@article{nk_connected_un_22, year = {2022}, journal = {INFORMS Journal on Optimization}, doi = {10.1287/ijoo.2021.0067}, author = {Nohadani, Omid and Sharma, Kartikey}, title = {Optimization Under Connected Uncertainty} }
- Tsuji, K., Tanaka, K., and Pokutta, S. (2022). Pairwise Conditional Gradients without Swap Steps and Sparser Kernel Herding. Proceedings of International Conference on Machine Learning.
[arXiv]
[summary]
[slides]
[code]
[video]
[BibTeX]
@inproceedings{ttp_pairwise_22, year = {2022}, booktitle = {Proceedings of International Conference on Machine Learning}, archiveprefix = {arXiv}, eprint = {2110.12650}, primaryclass = {math.OC}, author = {Tsuji, Kazuma and Tanaka, Ken'ichiro and Pokutta, Sebastian}, title = {Pairwise Conditional Gradients without Swap Steps and Sparser Kernel Herding}, code = {https://github.com/ZIB-IOL/FrankWolfe.jl}, slides = {https://pokutta.com/slides/20220624_ICML2022_BPCG.pdf}, summary = {https://pokutta.com/blog/research/2022/05/21/bpcg-abstract.html}, video = {https://slideslive.com/38983561} }
- Wäldchen, S., Huber, F., and Pokutta, S. (2022). Training Characteristic Functions with Reinforcement Learning: XAI-methods Play Connect Four. Proceedings of International Conference on Machine Learning.
[arXiv]
[poster]
[video]
[BibTeX]
@inproceedings{whp_connectfour_22, year = {2022}, booktitle = {Proceedings of International Conference on Machine Learning}, archiveprefix = {arXiv}, eprint = {2202.11797}, primaryclass = {cs.LG}, author = {Wäldchen, Stephan and Huber, Felix and Pokutta, Sebastian}, title = {Training Characteristic Functions with Reinforcement Learning: XAI-methods Play Connect Four}, poster = {https://pokutta.com/slides/20220712_icml_poster_conn4.pdf}, video = {https://slideslive.com/38983111} }
- Zimmer, M., Spiegel, C., and Pokutta, S. (2022). Compression-aware Training of Neural Networks Using Frank-Wolfe.
[arXiv]
[BibTeX]
@misc{zsp_deepsparsefw_22, archiveprefix = {arXiv}, eprint = {2205.11921}, primaryclass = {cs.LG}, year = {2022}, author = {Zimmer, Max and Spiegel, Christoph and Pokutta, Sebastian}, title = {Compression-aware Training of Neural Networks Using Frank-Wolfe} }
- Ziemke, T., Sering, L., Vargas Koch, L., Zimmer, M., Nagel, K., and Skutella, M. (2021). Flows Over Time As Continuous Limits of Packet-based Network Simulations. Transportation Research Procedia, 52, 123–130.
DOI: 10.1016/j.trpro.2021.01.014
[URL]
[BibTeX]
@article{zskzns_flows_21, year = {2021}, journal = {Transportation Research Procedia}, volume = {52}, pages = {123-130}, doi = {10.1016/j.trpro.2021.01.014}, url = {https://sciencedirect.com/science/article/pii/S2352146521000284}, author = {Ziemke, Theresa and Sering, Leon and Vargas Koch, Laura and Zimmer, Max and Nagel, Kai and Skutella, Martin}, title = {Flows Over Time As Continuous Limits of Packet-based Network Simulations} }
- Combettes, C., Spiegel, C., and Pokutta, S. (2020). Projection-free Adaptive Gradients for Large-scale Optimization.
[arXiv]
[summary]
[code]
[BibTeX]
@misc{csp_adafw_20, archiveprefix = {arXiv}, eprint = {2009.14114}, primaryclass = {math.OC}, year = {2020}, author = {Combettes, Cyrille and Spiegel, Christoph and Pokutta, Sebastian}, title = {Projection-free Adaptive Gradients for Large-scale Optimization}, code = {https://github.com/ZIB-IOL/StochasticFrankWolfe}, summary = {https://pokutta.com/blog/research/2020/10/21/adasfw.html} }
- Pokutta, S., Spiegel, C., and Zimmer, M. (2020). Deep Neural Network Training with Frank-Wolfe.
[arXiv]
[summary]
[code]
[BibTeX]
@misc{zsp_deepfw_20, archiveprefix = {arXiv}, eprint = {2010.07243}, primaryclass = {cs.LG}, year = {2020}, author = {Pokutta, Sebastian and Spiegel, Christoph and Zimmer, Max}, title = {Deep Neural Network Training with Frank-Wolfe}, code = {https://github.com/ZIB-IOL/StochasticFrankWolfe}, summary = {https://pokutta.com/blog/research/2020/11/11/NNFW.html} }