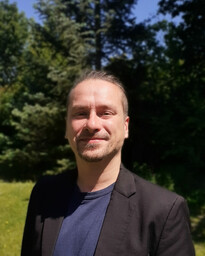
Patrick Gelß
My main area of focus lies in the development and application of quantum- and tensor-based methods. These methodologies have proven to be incredibly effective in solving complex problems across a range of fields. In addition to this, I have a strong interest in various topics in the fields of machine learning and data-driven approaches, kernel-based techniques, dynamical systems and transfer operators, catalytic systems, chemical reaction networks, and quantum mechanics, including quantum computing and simulation.
📬 Contact
- office
- Room 1356 at ZIB
- gelss (at) zib.de
p.gelss (at) fu-berlin.de - homepage
- patrickgelss.com
- languages
- German and English
🎓 Curriculum vitae
- since 2022
- Researcher at ZIB
- 2021 to 2022
- Researcher at FUB
- 2020 to 2020
- Researcher at ZIB
- 2017 to 2020
- Researcher at FUB
- Jun 2017
- Ph.D. in Applied Mathematics at FUB
- Oct 2013
- Diploma in Mathematics at FUB
📝 Publications and preprints
Preprints
- Stengl, S.-M., Gelß, P., Klus, S., and Pokutta, S. (2023). Existence and Uniqueness of Solutions of the Koopman–von Neumann Equation on Bounded Domains.
[arXiv]
[BibTeX]
@misc{KoopmanNeumann2023, archiveprefix = {arXiv}, eprint = {2306.13504}, primaryclass = {math.AP}, year = {2023}, author = {Stengl, Steven-Marian and Gelß, Patrick and Klus, Stefan and Pokutta, Sebastian}, title = {Existence and Uniqueness of Solutions of the Koopman--von Neumann Equation on Bounded Domains} }
- Gelß, P., Issagali, A., and Kornhuber, R. (2023). Fredholm Integral Equations for Function Approximation and the Training of Neural Networks.
[arXiv]
[BibTeX]
@misc{gik_fredholm_23, archiveprefix = {arXiv}, eprint = {2303.05262}, primaryclass = {math.NA}, year = {2023}, author = {Gelß, Patrick and Issagali, Aizhan and Kornhuber, Ralf}, title = {Fredholm Integral Equations for Function Approximation and the Training of Neural Networks} }
- Gelß, P., Klein, R., Matera, S., and Schmidt, B. (2023). Quantum Dynamics of Coupled Excitons and Phonons in Chain-like Systems: Tensor Train Approaches and Higher-order Propagators.
[arXiv]
[BibTeX]
@misc{gkms_tdse_23, archiveprefix = {arXiv}, eprint = {2302.03568}, primaryclass = {quant-ph}, year = {2023}, author = {Gelß, Patrick and Klein, Rupert and Matera, Sebastian and Schmidt, Burkhard}, title = {Quantum Dynamics of Coupled Excitons and Phonons in Chain-like Systems: Tensor Train Approaches and Higher-order Propagators} }
- Klus, S., and Gelß, P. (2023). Continuous Optimization Methods for the Graph Isomorphism Problem.
[arXiv]
[BibTeX]
@misc{kg_graph_isomorphism, archiveprefix = {arXiv}, eprint = {2311.16912}, primaryclass = {cs.DM}, year = {2023}, author = {Klus, Stefan and Gelß, Patrick}, title = {Continuous Optimization Methods for the Graph Isomorphism Problem} }
- Gelß, P., Klus, S., Shakibaei, Z., and Pokutta, S. (2022). Low-rank Tensor Decompositions of Quantum Circuits.
[arXiv]
[BibTeX]
@misc{gksp_lowrankqc_22, archiveprefix = {arXiv}, eprint = {2205.09882}, primaryclass = {quant-ph}, year = {2022}, author = {Gelß, Patrick and Klus, Stefan and Shakibaei, Zarin and Pokutta, Sebastian}, title = {Low-rank Tensor Decompositions of Quantum Circuits} }
- Gelß, P., and Schütte, C. (2018). Tensor-generated Fractals - Using Tensor Decompositions for Creating Self-similar Patterns.
[arXiv]
[BibTeX]
@misc{gs_fractals_18, archiveprefix = {arXiv}, eprint = {1812.00814}, primaryclass = {math.GM}, year = {2018}, author = {Gelß, Patrick and Schütte, Christof}, title = {Tensor-generated Fractals - Using Tensor Decompositions for Creating Self-similar Patterns} }
Full articles
- Designolle, S., Iommazzo, G., Besançon, M., Knebel, S., Gelß, P., and Pokutta, S. (2023). Improved Local Models and New Bell Inequalities Via Frank-Wolfe Algorithms. Physical Review Research, 5(4).
DOI: 10.1103/PhysRevResearch.5.043059
[arXiv]
[slides]
[code]
[BibTeX]
@article{dibkgp_bell_23, year = {2023}, journal = {Physical Review Research}, month = oct, volume = {5}, number = {4}, doi = {10.1103/PhysRevResearch.5.043059}, archiveprefix = {arXiv}, eprint = {2302.04721}, primaryclass = {quant-ph}, author = {Designolle, Sébastien and Iommazzo, Gabriele and Besançon, Mathieu and Knebel, Sebastian and Gelß, Patrick and Pokutta, Sebastian}, title = {Improved Local Models and New Bell Inequalities Via Frank-Wolfe Algorithms}, code = {https://github.com/ZIB-IOL/BellPolytopes.jl}, slides = {https://www.pokutta.com/slides/20230808-tokyo-bell.pdf} }
- Riedel, J., Gelß, P., Klein, R., and Schmidt, B. (2023). WaveTrain: A Python Package for Numerical Quantum Mechanics of Chain-like Systems Based on Tensor Trains. The Journal of Chemical Physics, 158(16), 164801.
DOI: 10.1063/5.0147314
[URL]
[arXiv]
[BibTeX]
@article{rgks_wavetrain_23, year = {2023}, journal = {The Journal of Chemical Physics}, volume = {158}, number = {16}, pages = {164801}, doi = {10.1063/5.0147314}, url = {https://pubs.aip.org/aip/jcp/article/158/16/164801/2887212/WaveTrain-A-Python-package-for-numerical-quantum}, archiveprefix = {arXiv}, eprint = {2302.03725}, primaryclass = {quant-ph}, author = {Riedel, Jerome and Gelß, Patrick and Klein, Rupert and Schmidt, Burkhard}, title = {WaveTrain: A Python Package for Numerical Quantum Mechanics of Chain-like Systems Based on Tensor Trains} }
- Gelß, P., Klein, R., Matera, S., and Schmidt, B. (2022). Solving the Time-independent Schrödinger Equation for Chains of Coupled Excitons and Phonons Using Tensor Trains. The Journal of Chemical Physics, 156, 024109.
DOI: 10.1063/5.0074948
[URL]
[arXiv]
[BibTeX]
@article{gkms_tise_22, year = {2022}, journal = {The Journal of Chemical Physics}, volume = {156}, pages = {024109}, doi = {10.1063/5.0074948}, url = {https://pubs.aip.org/aip/jcp/article/156/2/024109/2839835/Solving-the-time-independent-Schrodinger-equation}, archiveprefix = {arXiv}, eprint = {2109.15104}, primaryclass = {physics.comp-ph}, author = {Gelß, Patrick and Klein, Rupert and Matera, Sebastian and Schmidt, Burkhard}, title = {Solving the Time-independent Schrödinger Equation for Chains of Coupled Excitons and Phonons Using Tensor Trains} }
- Gelß, P., Klus, S., Schuster, I., and Schütte, C. (2021). Feature Space Approximation for Kernel-based Supervised Learning. Knowledge-Based Systems, 221, 106935.
DOI: 10.1016/j.knosys.2021.106935
[URL]
[arXiv]
[BibTeX]
@article{gkss_fsa_21, year = {2021}, journal = {Knowledge-Based Systems}, volume = {221}, pages = {106935}, doi = {10.1016/j.knosys.2021.106935}, url = {https://sciencedirect.com/science/article/abs/pii/S0950705121001982}, archiveprefix = {arXiv}, eprint = {2011.12651}, primaryclass = {stat.ML}, author = {Gelß, Patrick and Klus, Stefan and Schuster, Ingmar and Schütte, Christof}, title = {Feature Space Approximation for Kernel-based Supervised Learning} }
- Klus, S., Gelß, P., Nüske, F., and Noé, F. (2021). Symmetric and Antisymmetric Kernels for Machine Learning Problems in Quantum Physics and Chemistry. Machine Learning: Science and Technology, 2(4), 18958.
DOI: 10.1088/2632-2153/ac14ad
[URL]
[arXiv]
[BibTeX]
@article{kgnn_antisymmetric_21, year = {2021}, journal = {Machine Learning: Science and Technology}, volume = {2}, number = {4}, pages = {18958}, doi = {10.1088/2632-2153/ac14ad}, url = {https://iopscience.iop.org/article/10.1088/2632-2153/ac14ad}, archiveprefix = {arXiv}, eprint = {2103.17233}, primaryclass = {quant-ph}, author = {Klus, Stefan and Gelß, Patrick and Nüske, Feliks and Noé, Frank}, title = {Symmetric and Antisymmetric Kernels for Machine Learning Problems in Quantum Physics and Chemistry} }
- Nüske, F., Gelß, P., Klus, S., and Clementi, C. (2021). Tensor-based Computation of Metastable and Coherent Sets. Physica D: Nonlinear Phenomena, 427, 133018.
DOI: 10.1016/j.physd.2021.133018
[URL]
[arXiv]
[BibTeX]
@article{ngkc_metastable_2021, year = {2021}, journal = {Physica D: Nonlinear Phenomena}, volume = {427}, pages = {133018}, doi = {10.1016/j.physd.2021.133018}, url = {https://sciencedirect.com/science/article/abs/pii/S0167278921001755}, archiveprefix = {arXiv}, eprint = {1908.04741}, primaryclass = {math.NA}, author = {Nüske, Feliks and Gelß, Patrick and Klus, Stefan and Clementi, Cecilia}, title = {Tensor-based Computation of Metastable and Coherent Sets} }
- Gelß, P., Klus, S., Eisert, J., and Schütte, C. (2019). Multidimensional Approximation of Nonlinear Dynamical Systems. Journal of Computational and Nonlinear Dynamics, 14(6), 25094.
DOI: 10.1115/1.4043148
[URL]
[arXiv]
[BibTeX]
@article{gkes_mandy_19, year = {2019}, journal = {Journal of Computational and Nonlinear Dynamics}, volume = {14}, number = {6}, pages = {25094}, doi = {10.1115/1.4043148}, url = {https://asmedigitalcollection.asme.org/computationalnonlinear/article-abstract/14/6/061006/726935/Multidimensional-Approximation-of-Nonlinear}, archiveprefix = {arXiv}, eprint = {1809.02448}, primaryclass = {math.DS}, author = {Gelß, Patrick and Klus, Stefan and Eisert, Jens and Schütte, Christof}, title = {Multidimensional Approximation of Nonlinear Dynamical Systems} }
- Klus, S., and Gelß, P. (2019). Tensor-based Algorithms for Image Classification. Algorithms, 12(11), 240.
DOI: 10.3390/a12110240
[URL]
[arXiv]
[BibTeX]
@article{kg_imageclass_19, year = {2019}, journal = {Algorithms}, volume = {12}, number = {11}, pages = {240}, doi = {10.3390/a12110240}, url = {https://mdpi.com/1999-4893/12/11/240}, archiveprefix = {arXiv}, eprint = {1910.02150}, primaryclass = {cs.LG}, author = {Klus, Stefan and Gelß, Patrick}, title = {Tensor-based Algorithms for Image Classification} }
- Gelß, P., Klus, S., Matera, S., and Schütte, C. (2017). Nearest-neighbor Interaction Systems in the Tensor-train Format. Journal of Computational Physics, 341, 140–162.
DOI: 10.1016/j.jcp.2017.04.007
[URL]
[arXiv]
[BibTeX]
@article{gkms_slim_17, year = {2017}, journal = {Journal of Computational Physics}, volume = {341}, pages = {140-162}, doi = {10.1016/j.jcp.2017.04.007}, url = {https://sciencedirect.com/science/article/abs/pii/S0021999117302784}, archiveprefix = {arXiv}, eprint = {1611.03755}, primaryclass = {math.NA}, author = {Gelß, Patrick and Klus, Stefan and Matera, Sebastian and Schütte, Christof}, title = {Nearest-neighbor Interaction Systems in the Tensor-train Format} }
- Klus, S., Gelß, P., Peitz, S., and Schütte, C. (2017). Tensor-based Dynamic Mode Decomposition. Nonlinearity, 31(7), 3359.
DOI: 10.1088/1361-6544/aabc8f
[URL]
[arXiv]
[BibTeX]
@article{kgps_dmd_17, year = {2017}, journal = {Nonlinearity}, volume = {31}, number = {7}, pages = {3359}, doi = {10.1088/1361-6544/aabc8f}, url = {https://iopscience.iop.org/article/10.1088/1361-6544/aabc8f}, archiveprefix = {arXiv}, eprint = {1606.06625}, primaryclass = {math.NA}, author = {Klus, Stefan and Gelß, Patrick and Peitz, Sebastian and Schütte, Christof}, title = {Tensor-based Dynamic Mode Decomposition} }
- Gelß, P., Matera, S., and Schütte, C. (2016). Solving the Master Equation without Kinetic Monte Carlo - Tensor Train Approximations for a CO Oxidation Model. Journal of Computational Physics, 314, 489–502.
DOI: 10.1016/j.jcp.2016.03.025
[URL]
[BibTeX]
@article{gms_oxidation_16, year = {2016}, journal = {Journal of Computational Physics}, volume = {314}, pages = {489-502}, doi = {10.1016/j.jcp.2016.03.025}, url = {https://sciencedirect.com/science/article/abs/pii/S0021999116001777}, author = {Gelß, Patrick and Matera, Sebastian and Schütte, Christof}, title = {Solving the Master Equation without Kinetic Monte Carlo - Tensor Train Approximations for a CO Oxidation Model} }
- Mossner, M., Jann, J.-C., Wittig, J., Nolte, F., Fey, S., Nowak, V., Obländer, J., Pressler, J., Palme, I., Xanthopoulos, C., Boch, T., Metzgeroth, G., Röhl, H., Witt, S. H., Dukal, H., Klein, C., Schmitt, S., Gelß, P., Platzbecker, U., … Nowak, D. (2016). Mutational Hierarchies in Myelodysplastic Syndromes Dynamically Adapt and Evolve Upon Therapy Response and Failure. Blood, 128(9), 1246–1259.
DOI: 10.1182/blood-2015-11-679167
[URL]
[BibTeX]
@article{mjgmn_mutational_16, year = {2016}, journal = {Blood}, volume = {128}, number = {9}, pages = {1246-1259}, doi = {10.1182/blood-2015-11-679167}, url = {https://ashpublications.org/blood/article/128/9/1246/35782/Mutational-hierarchies-in-myelodysplastic}, author = {Mossner, Maximilian and Jann, Johann-Christoph and Wittig, Janina and Nolte, Florian and Fey, Stephanie and Nowak, Verena and Obländer, Julia and Pressler, Jovita and Palme, Iris and Xanthopoulos, Christina and Boch, Tobias and Metzgeroth, Georgia# and Röhl, Henning and Witt, Stephanie H. and Dukal, Helene and Klein, Corinna and Schmitt, Steffen and Gelß, Patrick and Platzbecker, Uwe and Balaian, Ekaterina and Fabarius, Alice and Blum, Helmut and Schulze, Torsten J. and Meggendorfer, Manja and Haferlach, Claudia and Trumpp, Andreas and Hofmann, Wolf-Karsten and Medyouf, Hind and Nowak, Daniel}, title = {Mutational Hierarchies in Myelodysplastic Syndromes Dynamically Adapt and Evolve Upon Therapy Response and Failure} }
🔬 Projects
Computational devices and quantum mechanics revolutionized the 20th century. Quantum computation merges these fields to solve optimization problems faster than classical computers. This project aims to develop new quantum algorithms for general-purpose and specific applications, including optimization, mixed-integer programs, and uses in machine learning, logistics, big data, and physics. We will explore quantum dynamic programming, graph sparsification, and QAOA-type algorithms for small quantum computers.
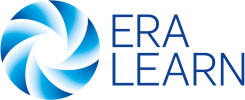
💬 Talks and posters
Conference and workshop talks
- Mar 2024
- Tensor-based Approaches for Modeling and Simulation of Molecular Systems
GAMM Conference - Feb 2024
- Fredholm Integral Equations for the Training of Shallow Neural Networks
7th SIAM UQ Conference - Sep 2023
- Fredholm Integral Equations for the Training of Shallow Neural Networks
7th RIKEN-MODAL Workshop, Berlin - Sep 2023
- Quantum Computing and Integer Programming
1st NHR Conference - Jun 2023
- Fredholm Integral Equations for the Training of Shallow Neural Networks
1st Workshop on Quantum Computation and Optimization, Berlin
View More / Less
- Mar 2023
- Solving Kinetic Master Equations with Tensor Trains
11th SIAM CSE Conference - Sep 2022
- Low-rank Tensor Decompositions of Quantum Circuits
6th RIKEN-MODAL Workshop, Tokyo / Fukuoka - Jun 2022
- Tensor-based Training of Neural Networks
24th ILAS Conference - May 2022
- Low-rank Tensor Decompositions of Quantum Circuits
3rd Workshop on Quantum Algorithms and Applications, Brussels - Dec 2019
- Tensor-based Algorithms for Image Classification
Mathematics of Deep Learning - Mar 2019
- The Tensor-train Format and Its Applications
89th GAMM Conference - Mar 2018
- Tensor-train Approximations for Catalytic Reaction Systems
DPG Spring Meeting of the Condensed Matter Section - Aug 2017
- Solving the Master Equation without Kinetic Monte Carlo
7th International Symposium on Energy - Mar 2017
- Solving the Master Equation without Kinetic Monte Carlo - Tensor Train Approximations for a CO Oxidation Model
DPG Spring Meeting of the Condensed Matter Section - Jan 2017
- SLIM-decomposition of Tensor Train Operators for Nearest-neighbor Interaction Networks
Model Reduction of Complex Dynamical Systems
Research seminar talks
- Jan 2024
- Tensor Decompositions
IBM QCS seminar Seminar, Zurich - Jul 2023
- Fredholm Integral Equations for the Training of Shallow Neural Networks
IBM NOIP seminar Seminar, Berlin - Jun 2023
- Fredholm Integral Equations for the Training of Shallow Neural Networks
DESY CQTA seminar Seminar, Zeuthen - Oct 2022
- The Tensor-train Format and Its Applications
Tensor Learning Team Seminar
👨🏫Teaching
- summer 2022
- Lecturer for high-dimensional discretization at FUB
- winter 2021
- Tutor for functional analysis at FUB
- summer 2020
- Tutor for dynamical systems at FUB
- winter 2019
- Lecturer for functional analysis at FUB
- summer 2019
- Lecturer for tensor decompositions and their applications at FUB
- winter 2018
- Tutor for functional analysis at FUB