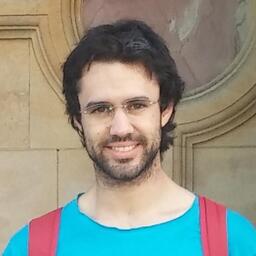
David Martínez-Rubio
postdoctoral researcher at ZIB since January 2022📬 Contact
office | Room 3107 at
ZIB Room MA 604 at TUB |
---|---|
martinez-rubio (at) zib.de | |
homepage | damaru2.github.io |
languages | English, Spanish, and Toki Pona |
🎓 Academic Background
Jan 2022 | Ph.D. in Computer Science at Oxford |
---|---|
Aug 2017 | M.Sc. in Mathematics and Foundations of Computer Science at Oxford |
Jul 2016 | B.Sc. in Computer Science and Engineering at UCM |
Jul 2016 | B.Sc. in Mathematics at UCM |
🔬 Research
Preprints
- Martínez-Rubio, D., Roux, C., and Pokutta, S. (2024). Convergence and Trade-offs in Riemannian Gradient Descent and Riemannian Proximal Point.
[arXiv]
[BibTeX]
@misc{mrp_tradeoffs_riemannian_gradient_descent_23, archiveprefix = {arXiv}, eprint = {2403.10429}, primaryclass = {math.OC}, year = {2024}, author = {Martínez-Rubio, David and Roux, Christophe and Pokutta, Sebastian}, title = {Convergence and Trade-offs in Riemannian Gradient Descent and Riemannian Proximal Point} }
- Scieur, D., Kerdreux, T., Martínez-Rubio, D., d’Aspremont, A., and Pokutta, S. (2023). Strong Convexity of Sets in Riemannian Manifolds.
[arXiv]
[BibTeX]
@misc{skmap_strong_convexity_of_sets_in_riemannian_manifolds_23, archiveprefix = {arXiv}, eprint = {2312.03583}, primaryclass = {math.OC}, year = {2023}, author = {Scieur, Damien and Kerdreux, Thomas and Martínez-Rubio, David and d'Aspremont, Alexandre and Pokutta, Sebastian}, title = {Strong Convexity of Sets in Riemannian Manifolds} }
Conference proceedings
- Criscitiello, C., Martínez-Rubio, D., and Boumal, N. (2023). Open Problem: Polynomial Linearly-convergent Method for G-convex Optimization? Proceedings of Annual Workshop on Computational Learning Theory.
[arXiv]
[BibTeX]
@inproceedings{cmb_open_problem_polynomial_linearly_convergent_method_for_gconvex_optimization_23, year = {2023}, booktitle = {Proceedings of Annual Workshop on Computational Learning Theory}, archiveprefix = {arXiv}, eprint = {2307.12743}, primaryclass = {math.OC}, author = {Criscitiello, Christopher and Martínez-Rubio, David and Boumal, Nicolas}, title = {Open Problem: Polynomial Linearly-convergent Method for G-convex Optimization?} }
- Martínez-Rubio, D., and Pokutta, S. (2023). Accelerated Riemannian Optimization: Handling Constraints with a Prox to Bound Geometric Penalties. Proceedings of Annual Workshop on Computational Learning Theory.
[arXiv]
[BibTeX]
@inproceedings{mp_acceleratedriemannian_22:1, year = {2023}, booktitle = {Proceedings of Annual Workshop on Computational Learning Theory}, archiveprefix = {arXiv}, eprint = {2211.14645}, primaryclass = {math.OC}, author = {Martínez-Rubio, David and Pokutta, Sebastian}, title = {Accelerated Riemannian Optimization: Handling Constraints with a Prox to Bound Geometric Penalties} }
- Martínez-Rubio, D., Roux, C., Criscitiello, C., and Pokutta, S. (2023). Accelerated Riemannian Min-Max Optimization Ensuring Bounded Geometric Penalties. Proceedings of Optimization for Machine Learning (NeurIPS Workshop OPT 2023).
[arXiv]
[BibTeX]
@inproceedings{mrp_accelerated_minmax_riemannian_23, year = {2023}, booktitle = {Proceedings of Optimization for Machine Learning (NeurIPS Workshop OPT 2023)}, archiveprefix = {arXiv}, eprint = {2305.16186}, primaryclass = {math.OC}, author = {Martínez-Rubio, David and Roux, Christophe and Criscitiello, Christopher and Pokutta, Sebastian}, title = {Accelerated Riemannian Min-Max Optimization Ensuring Bounded Geometric Penalties} }
- Martínez-Rubio, D., Wirth, E., and Pokutta, S. (2023). Accelerated and Sparse Algorithms for Approximate Personalized PageRank and Beyond. Proceedings of Annual Workshop on Computational Learning Theory.
[arXiv]
[BibTeX]
@inproceedings{mwp_accelerated_sparse_pagerank_23, year = {2023}, booktitle = {Proceedings of Annual Workshop on Computational Learning Theory}, archiveprefix = {arXiv}, eprint = {2303.12875}, primaryclass = {math.OC}, author = {Martínez-Rubio, David and Wirth, Elias and Pokutta, Sebastian}, title = {Accelerated and Sparse Algorithms for Approximate Personalized PageRank and Beyond} }
- Criado, F., Martínez-Rubio, D., and Pokutta, S. (2022). Fast Algorithms for Packing Proportional Fairness and Its Dual. Proceedings of Conference on Neural Information Processing Systems.
[arXiv]
[poster]
[BibTeX]
@inproceedings{cmp_packing_proportional_fairness_22, year = {2022}, booktitle = {Proceedings of Conference on Neural Information Processing Systems}, archiveprefix = {arXiv}, eprint = {2109.03678}, primaryclass = {math.OC}, author = {Criado, Francisco and Martínez-Rubio, David and Pokutta, Sebastian}, title = {Fast Algorithms for Packing Proportional Fairness and Its Dual}, poster = {https://pokutta.com/slides/20211105_fairpacking-poster.pdf} }
- Martínez-Rubio, D., and Pokutta, S. (2022). Accelerated Riemannian Optimization: Handling Constraints with a Prox to Bound Geometric Penalties. Proceedings of Optimization for Machine Learning (NeurIPS Workshop OPT 2022).
[URL]
[arXiv]
[poster]
[BibTeX]
@inproceedings{mp_acceleratedriemannian_22, year = {2022}, booktitle = {Proceedings of Optimization for Machine Learning (NeurIPS Workshop OPT 2022)}, url = {https://opt-ml.org/papers/2022/paper27.pdf}, archiveprefix = {arXiv}, eprint = {2211.14645}, primaryclass = {math.OC}, author = {Martínez-Rubio, David and Pokutta, Sebastian}, title = {Accelerated Riemannian Optimization: Handling Constraints with a Prox to Bound Geometric Penalties}, poster = {https://pokutta.com/slides/20221203_poster_neurips_riemannian.pdf} }